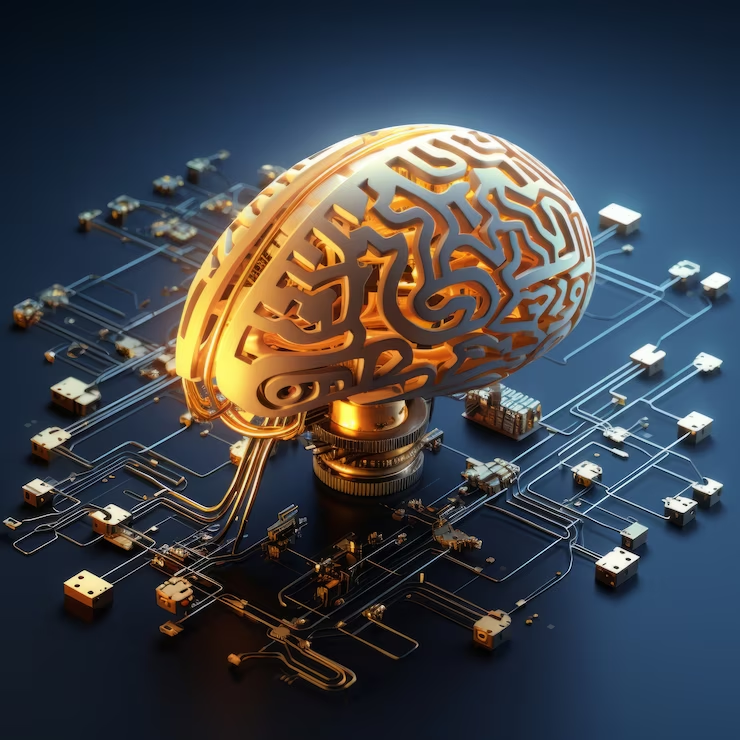
Explore the cutting-edge world of AI development with insights into the most popular challenges and practices. Stay ahead of the curve inartificial intelligence as we delve into industry trends, best practices, and emerging challenges shaping the future of AI development.
AI development is already a commonplace term in the computer sector and has a significant impact on our daily lives. Artificial Intelligence is pervasive, ranging from voice assistants such as Siri and Alexa to tailored suggestions on Netflix and Amazon. If you’re the founder of a firm, you may be curious about the difficulties presented by artificial intelligence (AI) and how you can use it to enhance your operations, products, and data to remain one step ahead of the competition.
Top Challenges with Artificial Intelligence
If you’re considering developing AI software for your business, there will probably be some challenges. Knowing them in advance might make your task easier. The following are the most typical issues with details with AI development and applications that you may run across:
The Challenge of Data Quality and Availability
AI solutions face challenges in data availability and quality, which can hinder their effectiveness. To overcome this, businesses must invest in data management and quality, ensuring accurate, comprehensive, and relevant information. Techniques like data normalization, enrichment, and cleaning can help, and businesses should also consider adding additional data sources to their internal data.
Talent Shortage and Skill Gap
Employers often bring on new hires to handle AI-related duties. Retraining and upskilling current staff members, as well as providing thorough training on artificial intelligence (AI) and the potential it presents to businesses, is, nonetheless, considerably more advised and far less dangerous. Numerous artificial intelligence courses are available for free, including this one from Helsinki University.
Overcoming Bias and Fairness Challenges
High-quality data is crucial for AI system success, and biases in training data or algorithm output can hinder AI deployment. Prejudices based on race, gender, and community/ethnicity can also contribute to low-quality data. To overcome these biases, simple algorithms and impartial datasets should be used. Artificial intelligence development businesses invest in control frameworks and methods to enhance transparency and trust in AI systems.
AI Integration
The main challenge in deploying AI in business is integrating AI into existing systems, which requires expert assistance from AI solution providers. The transition requires careful consideration of data intake, storage, and infrastructure, ensuring seamless system functionality and compliance with AI regulations. Post-changeover, staff must receive the necessary training to operate the new system.
Absence of Comprehension
One drawback is that executives often overlook the possibilities and the revolutionary effect artificial intelligence (AI) may have on a business. Given that a company’s options for tools and AI technologies are always expanding, it is critical to understand what options are accessible. Managers should also consider the best times to execute various AI projects and have a critical mentality in order to identify which AI initiatives are most important and should be adopted first.
Best Practices for Successful AI Development
37% of companies have already used AI services as of 2022 and are still doing so. Research predicts that by 2025, the AI sector will generate $126 billion in revenue annually. In order for it to generate income for you, it is important to familiarize yourself with a description of the best practices for using artificial intelligence.
Train Your AI Models
An ML model needs high-quality historical data to be trained. Using the Auto Machine Learning engine, create voice, images, videos, and natural language recognition (AutoML). Using a drag-and-drop interface, the AutoML engine enables users to submit photos and have ML models generated automatically. Train the model, import the data, and label the data. The finest thing is that all of the labor-intensive work is handled by the AutoML engine.
Choosing the Right AI Algorithms
AI algorithms analyze large data volumes to derive valuable insights. They come in various forms, such as deep learning for voice and picture recognition and decision trees for classification. The choice of algorithm depends on the job, accuracy level, and dataset complexity. Some algorithms are better suited for complex datasets, while others are better for simple structures. Optimizing the algorithm for specific uses can increase performance and accuracy. Continuous improvement is crucial for optimal results.
Ensuring Deployment and Scalability in AI Models
Before implementing AI systems, it’s essential to plan for infrastructure needs, manage upgrades and changes, integrate with existing systems, ensure scalability through horizontal and vertical scaling, and use techniques like load balancing, caching, and asynchronous processing for enhanced performance. Constant monitoring and performance testing are crucial for effective growth.
Conclusion
Understanding the difference between AI and human intellect is crucial for preparing for the impact of AI on our lives. AI solves labor issues and recruitment costs for multinational corporations. Best practices for deploying AI include evaluating IT infrastructure, identifying use cases, understanding data, providing training, and tracking records. A long-term vision is also necessary.
Was this news helpful?